Snapshot data and longitudinal qualitative studies
In the last blog post, we looked at creating archives of qualitative data that can be used by other researchers (or yourself in the future) for secondary analysis. In that article I postulated that secondary data analysis could make collecting new data a rarer, and expensive event
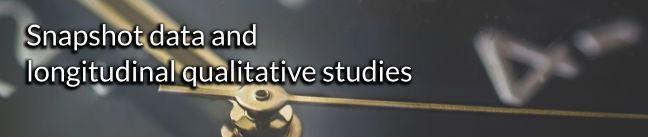
In the last blog post, we looked at creating archives of qualitative data that can be used by other researchers (or yourself in the future) for secondary analysis. In that article I postulated that secondary data analysis could make collecting new data a rarer, and expensive event. However, some (including Dr Susanne Friese) pointed out that as the social world is always changing, there is a constant need to collect new data. I totally agree with this, but it made me think about another problem with research studies – they are time-limited collection events that end up capturing a snapshot of the world when they were recorded.
Most qualitative research collects data as a series of one-off dives into the lives and experience of participants. These might be a single interview or focus group, or the results of a survey that captures opinions at a particular point in time. This might not be a certain date, it might also be at a key point in a participant’s journey – such as when people are discharged from hospital, or after they attend a training event. However, even within a small qualitative research project it is possible to measure change, by surveying the people at different stages in their individual or collective journeys.
This is sometimes called ‘Qualitative Longitudinal Research’ (Farrall et al. 2006), and often involves contacting people at regular intervals, possibly even after years or decades. Examples of this type of project include the five year ‘Timescapes’ project in the UK which looked at changes in personal and family relationships (Holland 2011), or a mutli-year look at homelessness and low-income families in Worcester, USA for which the archived data is available (yay!).
However, such projects tend to be expensive, as they require having researchers working on a project for many years. They also need quite a high sample size because there is an inevitable annual attrition of people who move, fall ill or stop responding to the researchers. Most projects don’t have the luxury of this sort of scale and resources, but there is another possibility to consider: if it is appropriate to collect data over a shorter timescale. This is often collecting data at ‘before and after’ data points – for example bookending a treatment or event, so are often used in well planned evaluations. Researchers can ask questions about expectations before the occasion, and how they felt afterwards. This is useful in a lot of user experience research, but also to understand motivations of actors, and improve delivery of key services.
But there are other reasons to do more than one interview with a participant. Even having just two data points from a participant can show changes in lives, circumstances and opinions, even when there isn’t an obvious event distinguishing the two snapshots. It also gets people to reflect on answers they gave in the first interview, and see if their feelings or opinions have changed. It can also give an opportunity to further explore topics that were interesting or not covered in the first interview, and allow for some checking – do people answer the questions in a consistent way? Sometimes respondents (or even interviewers) are having a bad day, and this doubles the chance of getting high quality data.
In qualitative analysis it is also an opportunity to look through all the data from a number of respondents and go back to ask new questions that are revealed from the data. In a grounded theory approach this is very valuable, but it can also be used to check researcher’s own interpretations and hypothesis about the research topic.
There are a number of research methods which are particularly suitable to longitudinal qualitative data studies. Participant diaries for example can be very illuminating, and can work over long or short periods of time. Survey methods also work well for multiple data points, and it is fairly easy to administer these at regular intervals by collecting data via e-mail, telephone or online questionnaires. These don’t even have to have very fixed questions, it is possible to do informal interviews via e-mail as well (eg Meho 2006), and this works well over long and medium periods of time.
So when planning a research project it’s worth thinking about whether your research question could be better answered with a longitudinal or multiple contact approach. If you decide this is the case, just be aware of the possibility that you won’t be able to contact everyone multiple times, and if means that some people’s data can’t be used, you need to over-recruit at the initial stages. However, the richer data and greater potential for reliability can often outweigh the extra work required. I also find it very illuminating as a researcher, to talk to participants after analysing some of their data. Qualitative data can become very abstract from participants (especially once it is transcribed, analysed and dissected) and meeting the person again and talking through some of the issues raised can help reconnect with the person and story.
Researchers should also consider how they will analyse such data. In qualitative analysis software like Quirkos, it is usually fairly easy to categorise the different sources you have by participant or time scale, so that you can look at just 1st or 2nd interviews or all together. I have had many questions from users into how best to organise their projects for such studies: and my advice is generally to keep everything in one project file. Quirkos makes it easy to do analysis on just certain data sources, and show results and reports from everything, or just one set of data.
So consider giving Quirkos a try with a free download for Windows, Mac or Linux, and read about how to build complex queries to explore your data over time, or between different groups of respondents.