Structuring unstructured data
The terms ‘unstructured data’ and ‘qualitative data’ are often used interchangeably, but unstructured data is becoming more commonly associated with data mining and big data approaches to text analytics. Here the comparison is drawn with
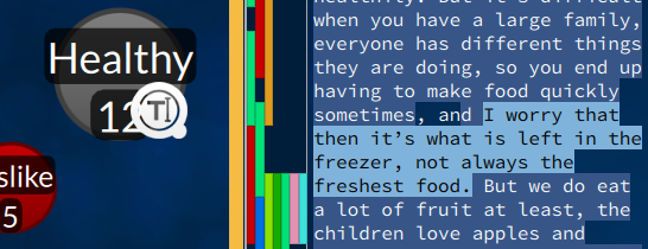
The terms ‘unstructured data’ and ‘qualitative data’ are often used interchangeably, but unstructured data is becoming more commonly associated with data mining and big data approaches to text analytics. Here the comparison is drawn with databases of data where we have a defined field and known value and the loosely structured (especially to a computer) world of language, discussion and comment. A qualitative researcher lives in a realm of unstructured data, the person they might be interviewing doesn’t have a happy/sad sign above their head, the researcher (or friend) must listen and interpret their interactions and speech to make a categorisation based on the available evidence.
At their core, all qualitative analysis software systems are based around defining and coding: selecting a piece of text, and assigning it to a category (or categories). However, it is easy to see this process as being ‘reductionist’: essentially removing a piece of data from it’s context, and defining it as a one-dimensional attribute. This text is about freedom. This text is about liberty. Regardless of the analytical insight of the researcher in deciding what relevant themes should be, and then filtering a sentence into that category, the final product appears to be a series of lists of sections of text.
This process leads to difficult questions such as, is this approach still qualitative? Without the nuanced connections between complicated topics and lived experiences, can we still call something that has been reduced to a binary yes/no association qualitative? Does this remove or abstract researchers from the data? Isn't this a way of quantifying qualitative data?
While such debates are similarly multifaceted, I would usually argue that this process of structuring qualitative data does begin to categorise and quantify it, and it does remove researchers from their data. But I also think that for most analytical tasks, this is OK, if not essential! Lee and Fielding (1996) say that “coding, like linking in hypertext, is a form of data reduction, and for many qualitative researchers is an important strategy which they would use irrespective of the availability of software”. When a researcher turns a life into 1 year ethnography, or a 1 hour interview, that is a form of data reduction. So is turning an audio transcript into text, and so is skim reading and highlighted printed versions of that text.
It’s important to keep an eye on the end game for most researchers: producing a well evidenced, accurate summary of a complex issue. Most research, as a formula to predict the world or a journal article describing it, is a communication exercise that (purely by the laws of entropy if not practicality) must be briefer than the sum of it’s parts. Yet we should also be much more aware that we are doing this, and together with our personal reflexivity think about the methodological reflexivity, and acknowledge what is being lost or given prominence in our chosen process.
Our brains are extremely good at comprehending the complex web of qualitative connections that make everyday life, and even for experienced researchers our intuitive insight into these processes often seem to go beyond any attempt to rationalise them. A structuralist approach to qualitative data can not only help as an aide-mémoir, but also to demonstrate our process to others, and challenge our own assumptions.
In general I would agree with Kelle (1997) that “the danger of methodological biases and distortion arising from the use of certain software packages is overemphasized in current discussions”. It’s not the tool, it’s how you use it!