Managing qualitative coding systems
In this blog post, I'm going to try and persuade you to do something really boring. Develop a formal process to record your analysis journey, and the coding systems you have.
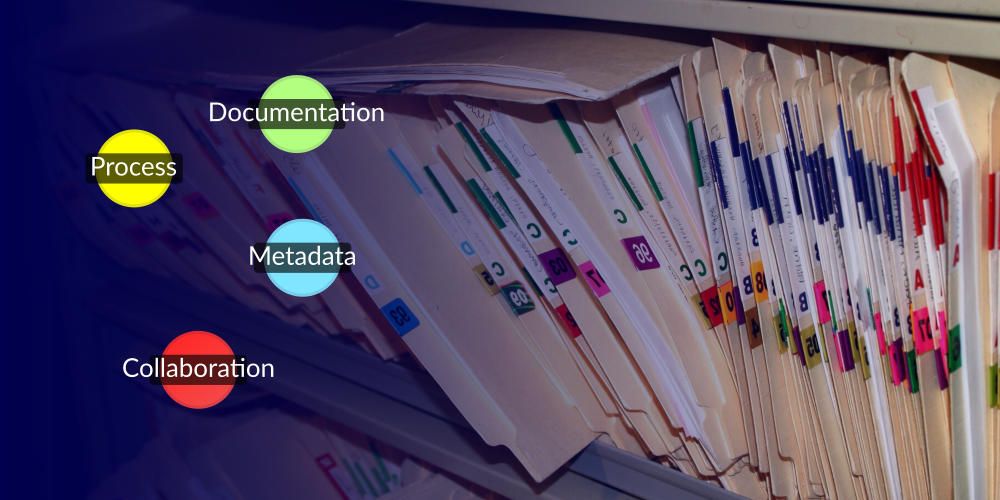
Unstructured qualitative data is amazing and rich, and one of the challenges with qualitative analysis is finding a way to help find a way to find and communicate the interesting things in the data. Coding is only one way to do this, but sometimes a coding framework can become as chaotic and unstructured as the data itself, representing the complexities of the underlying data and issues. If you are not careful, the coding framework itself can become an impediment to understanding the data, rather than a tool to help unravel it.
In this blog post, I'm going to try and persuade you to do something really boring. Develop a formal process to record your analysis journey, and the coding systems you have. Most people get really excited when you get the data in front of you, ready to read and explore it, and are keen to jump straight into the analysis. But eventually we all have to write up the data, and having a good process before you start will really help that. This requires a little planning, and taking the time to note down what you are doing and why.
So to mitigate against the seeming tedium of this process, I want to emphasise that the aim of this is to allow the coding and analysis process to get out of the way, so you can focus on being creative, experimental and getting into the data. It's not structure for structure's sake, but should be a way to help with the process, and save you time later on.
Meta
The most important concept for analysis systems is what would technically be called ‘meta-data’, that is data about the data itself. If you look at the article about codebooks, almost all the categories there are meta-data: information about the codes themselves. Who created it, what it's for, what kind of analysis it was for, when in the process it was created. That's all information that helps you keep track of all whole analysis process, something that can take months and have many shifts and changes in direction and fortune.
At the end of this process, you'll have to write up the analysis and describe what you did, and having all this meta-information is really important to being able to do this quickly and accurately. You may think you know the data really well, but there will be a point in your hundreds of codes and themes that you go 'Why did I create this?' and the more records you have of this process the easier understanding all this will be.
Choosing an appropriate system
You don’t need to use any software to manage this process, a paper method can be just as effective: the key thing is to make sure it’s a system that you are comfortable using, and keeping up-to-date. There’s no point adopting some fancy technological solution if it’s not how you like to work. I still have a paper notebook for all the work and projects I do, and I run a software company!
You can keep something as simple as a Word document or Excel spreadsheet as a research diary. These are easy to update and work with, and familiar for many users. Qualitative data analysis software also has specific tools and structures that can help; first of all it will keep a log of when most actions were performed, and have features like notes, memos and even chat in Quirkos Cloud that can let you document your metadata and management system. You can also le
Collaborating for Analysis
The above systems and procedures are essentially methods of communicating with yourself in the future - keeping records for writing up that make sure you remember the analytic process. However, these same systems are almost vital when collaborating with others.
Collaborative analysis is really useful for many reasons, for sharing the workload, validation or teaching. But when working as part of a team, it's vital to have clear guidelines so that everyone understands things like what they are supposed to code and how to access the data. Each person needs to understand the research questions, and for the coding process, what the type of analysis is - for example if you are doing thematic, discourse, open/axial, grounded theory etc. You also need to have a codebook that describes each of the codes, with detailed descriptions and examples of when to code. There also needs to be ways to update the codebooks, record questions and issues, and have regular review sessions so coders can discuss the coding and update the process.
This is especially important with grounded theory and other types of emergent analysis, where researchers will be creating new codes as they go along. There may need to be discussions about which ones to keep, or similar themes that can be merged, decisions that will change as the analysis progresses. Framework analysis is often easier for collaborative coding as the codes don't change as much, but it's rare that the coding framework doesn't get changed or refined at all during the process.
Quirkos Cloud was specifically designed to facilitate all kinds of collaborative analysis, and allows any number of users to work on a project at the same time. It also has a chat function, which is really useful for discussing issues, asking questions and recording notes on the process.
There's more on this in the chapter I wrote for the excellent textbook 'Analyzing and Interpreting Qualitative Research' which has fantastic contributions from amazing authors like Johnny Saldana, Charles Vanover, Paul Mihas, Jessica Lester, Trena Paulus, Silvana di Gregorio and so many more! There's a link for the texbook below below, but also a video interview and overview on the chapter:
