NOT Coding Qualitative Data, and Delayed Categorisation

When you finally get qualitative data in your hands, after planning, ethics approval, sampling and recruitment, collecting your data and getting it transcribed, it’s an exciting, and long-awaited moment. It's almost impossible to resist the temptation to jump straight into coding and exploring this intriguing and rich data. We’ve already talked about some reasons to stop and read your data before you start coding it, but this article is suggesting you try something different and difficult: delay coding your data as long as possible.
Of course, it’s not necessary in qualitative research to do any formal coding at all: many academics advocate just reading/listening to your data to become immersed in it, and see any form of coding or thematic analysis as reductive and diminishing the depth of qualitative data (eg St. Pierre et al. 2014). However, most researchers do some type of coding and analysis – it can help you keep track of all the many different facets in the data, and greatly helps writing up as you can quickly find, compare and include quotations from the data in your writing. Additionally, several approaches such as grounded theory, and constant comparative analysis require you to start analysing your data early, to inform sampling of other participants, and further refine interview questions. Even here analysis doesn’t have to mean coding, but I am proposing something that can still work with those approaches.
Whenever we are doing inductive coding / classic grounded theory, we are creating codes inspired by our reading and interpreting of the data. Note the ‘themes don’t emerge’ debate – they are not inherently present in qualitative data, the codes and words are actively chosen and shaped by the researcher who creates and frames them.
Imagine you are reading through your transcript, and someone says something interesting. You want to capture and categorise it! It might even seem obvious what they are talking about, so you create a code and assign that section of text to it (whether you are using software, or pen and paper). But whatever word or phrase you used to define that code is now crystallised, a code inspired by one interpretation of one piece of data. Later in the transcript, we might want to use it again, and make a conscious choice to add another piece of text to that code, even if it means something slightly different. And when we come to code another participants data, we will likely use codes from the first transcript, sometimes shoehorning meaning into a code that was created for another participant in a different context.
OK, this sounds a little dramatic. We can always change the name of the code, and often will do this as the code develops (and later may become part of an emerging theme), or split and merge codes with similar (or increasingly different) topics. It’s important to recognise that open-ended codes in grounded theory should be constantly evolving as we better understand the data, and place more data under them.
But still, that first word (or phrase) that popped into our head when we created that code has a legacy, and an influence not just on how we use that code, but how we read the data. Once I’ve created a code for ‘Anxiety’, I’m assessing whether data belongs in that ‘Anxiety’ code, as well as considering each piece of text against that code as the baseline for a different code I might want to create later called ‘Worry’ or ‘Fears’. So that code name has had a possibly discreet, but significant influence on all of the subsequent data we coded and interpreted.
Of course, other writers have commented on this problem before. I’d also argue that it connects with Derrida’s notion of deconstruction: very simply, an attempt to critique and problematise the meaning we assume to be associated with words. Derrida describes how the meaning of words is socially constructed, fluid, and difficult to define without using other words, which will have their own problematic interpretations. (Sediq 2024 has a good summary). In qualitative analysis, the labels and words we use for codes are imbude with our own meaning, and set in stone an interpretation that is not of the text, but above the text.
We’ve talked about some alternatives to coding before, including using emojis to create more pictorial representations of codes and themes. However, this also has many of the same issues with text coding, including that emoji have different meanings for different people.
But I’m proposing a different approach: try and go as long as you can without naming your codes! Try and make your approach a bit more like using coloured highlighters – you have a limited number of them, and sometimes you just have to code what’s interesting, rather than having a lot of discrete ‘categories’ or ‘categories’ represented by different colours. And most importantly, in the early stages these colours don't yet have labels.
The aim is to start highlighting interesting pieces of text, but without letting my brain start naming or categorising what I’m seeing. It sounds difficult to define (and it is), but I am constantly reminded of this direction to the player in a piano piece by Eric Satie: ‘du bout de la pensée’ (from Gnossienne no. 1). You can translate this a lot of ways, but I’ve always read it as ‘about the edge of an idea’ – nearly that half-daydreaming unconscious thinking when you are just about to have an idea, and can see the outline or shadow, but the idea itself is not yet formed. In Gnossienne, I think Satie is trying to get the player to be a little hesitant, but not shy, as if something is just about to click, if they don’t think about it too hard. One of the other directions in the piece is to play the melody in a way that is ‘questionnez’ (questioning), again a perfect but more direct mood for qualitative analysis.
So I tried this approach in Quirkos! I took a transcription of a semi-structured interview (from the open data set Qualitative Journeys Project) and eventually created three bubbles/codes with different colours, but didn’t give any of them a name. Then I went through and coded text to them that were roughly about something similar under each colour. Three codes seemed to be all I could really remember in my mind while going through them. I tried really hard to not have a ‘shortcut’ word in my head for what each meant, and tried to keep my thinking as open as possible: this is this sort of thing, or that sort of thing...
It was difficult, but in the end three themes sort of congregated together, I later described them as being quite broad areas, that were really open and interesting. The dataset was about qualitative careers in academia, and there was a theme (yes, they seemed much higher level than codes) about… institutional level issues in academia... one about career pathways... and one roughly about about things holding back qualitative research... A lot of sections of text were 'coded' to more than one code, but that was fine too:
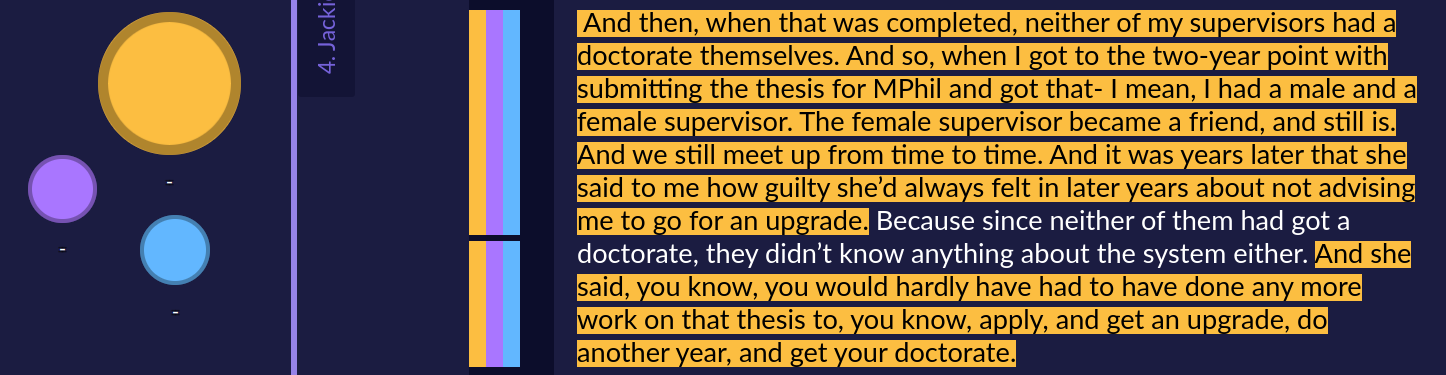
At this stage, I would have loved to keep going using the same ‘codes’ in other interviews, just to see how well they fit with other participants data. I didn’t get time to do this, but get the feeling that they were open and vague enough that it would have worked great – much better than trying to shoehorn very explicitly named codes like ‘Institutional problems’. This approach gave them space to evolve and grow naturally, without me having to worry about which way they were growing, or if the labeling was right.
Of course, this likely wouldn’t be enough analysis to be able to start writing up or drawing too many conclusions. But that’s OK – most of the time people need to go through their qualitative data more than once, especially with approaches like open/axial coding. But going through all the data first with a few very broad and non-defined themes, then revisiting the data with a more detailed (and labelled) approach seems to me a great way to let the data speak, and keep your own categorisations of the data at bay.
We’ve recently updated Quirkos Web to help with this approach. While you never had to give codes a name before, they would show as ‘untitled’ which annoyed me. Now they can just be left totally blank, and will stay just as coloured bubbles. Of course, you don’t need to use software for this kind of ‘no-coding’ approach, but software is so useful for later stages, and makes it easy to later split, merge and combine these codes quickly, especially with our revamped overview/recording view. So give Quirkos a try for free now, it works directly in your browser and makes qualitative analysis engaging and creative!
References:
Sediq, Mohammad. (2024). Unravelling Deconstruction: A Comprehensive Examination of its Qualitative Research Method and Application in Historical Texts. Sprin Journal of Arts, Humanities and Social Sciences. 3. 5-9. 10.55559/sjahss.v3i1.210.
St. Pierre, E. A., & Jackson, A. Y. (2014). Qualitative data analysis after coding. In: Sage Publications Sage CA: Los Angeles, CA.
Thanks to Lyn Lavery for inviting me to give a talk at the Research Accelerator 2023 conference on prototypes of these ideas, and for giving wonderful suggestions and feedback. Thanks also to all the great questions from this talk at the QHRN 2024 Workshop series!