Should you use inter-rater reliability in qualitative coding?
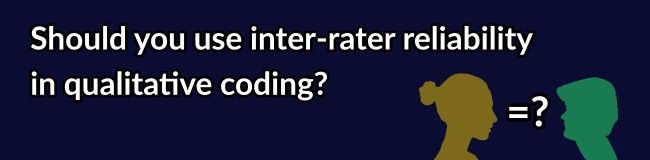
In qualitative analysis it’s sometimes difficult to agree even with yourself. With complex data sets, and ‘wicked’ issues, there are times that a researcher coding qualitative data will not consistently code different sources to the same themes or codes in the same way. Often there many themes, rich and numerous sources, and difficult decisions to be made as to where sections of text fit. So to promote consistency, researchers often take a cyclical approach to coding.
However, some researchers aim for better accuracy and consistency by having multiple people code the data, and check that they are making the same interpretations. Some would argue that this mitigates the subjectivity of a single coder/interpreter, producing a more valid and rigorous analysis (a very positivist interpretation). But multiple coders can also check each other’s work, and use differences to spark a discussion about the best way to interpret complex qualitative data. This is essentially a triangulation process between different researchers interpretations and coding of qualitative data.
Often, researchers will be asked to quantify the level of agreement between the different codes, looking at how often the coding agrees : that is usually that two (or more) coders have applied the same code to the same section of the data. There are a couple of different ways to calculate and measure agreement, the simplest being percentages, ratios or joint-probability of agreement. Cohen’s Kappa and Fleiss’s Kappa are two statistical tests often used in qualitative research to demonstrate a level of agreement. The basic difference is that Cohen’s Kappa is used between two coders, and Fleiss can be used between more than two. However, they use different methods to calculate ratios (and account for chance), so should not be directly compared.
All these are methods of calculating what is called ‘inter-rater reliability’ (IRR or RR) – how much raters agree about something. These tests are very common in psychology where they are used for having multiple people give binary diagnostics (positive/negative diagnoses), or delivering standardised tests – both situations that are probably better suited to measures of Kappa than qualitative coding.
But before qualitative researchers use any method of interrater reliablity, they should understand what they are and how they work. The first question should be: What are you looking to test? Is it that multiple researchers created the same code in a grounded theory approach? How often codes were used? How many of the highlights/quotes were coded in the same way? Each of these situations can be tested for IRR, but the latter is the most common.
Also, note that that when we are looking for agreement, there is an important difference between precious and accuracy:
“If we actually hit the bull’s-eye, we are accurate. If all our shots land together, we have good precision (good reliability). If all our shots land together and we hit the bull’s-eye, we are accurate as well as precise. It is possible, however, to hit the bull’s-eye purely by chance.” (Viera et al. 2005).
So be aware that just because two coders interpreted text the same way, it doesn’t mean they were both correct (they were reliable, but inaccurate).
It’s also important to realise that while the two different Kappa measurements have some control for chance and probability, they will be affected by sample size. And as always, the type and amount of data entered in to the test will affect the outcome and significance. Some qualitative software tools will give agreement figures (% or kappa) to two decimal places, and unless you have very large projects, this level of precision can be meaningless and misleading.
MacDonald et al. 2019 notes that “RR can be confusing because it merges a quantitative method, which has roots in positivism and objective discovery, with qualitative methods that favor an interpretivist view of knowledge”. I personally feel that it is methodologically incorrect to try and quantify qualitative data or the process behind it. Unless there is a very specific, tested and validated reason to do so, qualitative methods and data should not be analysed with quantitative tools.
So why are people so desperate for these quantitative metrics? The most common source of queries on this topic are posted by students or academics who have been demanded to demonstrate their coding is robust, by showing a Kappa figure of 0.8 or higher. These requests could come from supervisors, examiners or journal reviewers who have a quantitative background, and little understanding of qualitative methods and analysis. It has become a shortcut to demonstrate quality of research and that data was interpreted without bias.
MacDonald et al. 2019 again: “Quantitative researchers have sometimes made the mistake of evaluating qualitative research reports using the standards of quantitative research, expecting IRR regardless of the nature of the qualitative research. As a result, reporting statistical measures may be alluring for qualitative researchers who believe that reviewers who are unfamiliar with their methods will respond to IRR as a signal of reliability; however, for many methods, reliability measures and IRR don’t make sense. They may even be outright harmful.”
However, a statistician would not accept results from a test performed by someone who did not understand how the test was performed, and the influence of parameters on it. Nor would we be happy with statistical word-frequency analysis on qualitative data where the question and methodology demanded in-depth qualitative reading and analysis. In many situations, the quantification of qualitative coding in this way is not the right way to understand the data or analysis process.
Plus it could be argued that these processes are testing more the process, rather than the results. They are actually testing how well the raters know and apply the guidelines, and coding framework. There is a lot of work required to allow multiple coders to analyse a dataset, they need to understand what is meant by each code and when to apply it. This may create a coding manual or part of a detailed description between researchers. Good planning and preparation will go a long way to improve the accuracy and reliability of the process. This recent blog article talks about some of the other practical considerations for collaborating on the analysis of qualitative data.
So what do we do? I would argue we should identify, embrace and understand these differences. The bits of interpretation that we don’t agree on are probably the most interesting, just as with differences in opinions from our respondents. Why did people use different codes there? Why did people not code that the same way? Are there a few random outliers, or does it show a systematic difference in interpretation? We may want to embrace those differences, understanding that the networked lives and differences in experience or background of researchers is a vital tool in making better understanding of the complex lived experience in qualitative research. We need to recognise and acknowledge our subjectivity, and accept that for the questions that qualitative research asks, and the ways we interpret them, there may not always be a singular ‘correct’ way to code – no gold standard to aim for.
Perhaps the uncomfortable truth is that doing collaborative coding and aiming for ‘reliability’ actually requires an additional huge time input. Not only do you need to double the coding time, you also need to set aside the time to discuss, understand and ‘compromise’ all the differences in coding. A single quantitative metric gives a comfort blanket of quality without understanding what lies beneath it, and how it may be inaccurate. So if researchers get a 0.8 or higher kappa ratio, they can just move on and publish without having to think about why there were differences. Any button in qualitative software that throws out a figure for you, without letting you understand and change how it was calculated can be misused (even if well meaning). It’s not a shortcut for explaining to a supervisor or journal reviewer that demanding a particular Kappa figure is likely wrong, and inappropriate for many qualitative research projects (see MacDonald et al. 2019).
This is why we have deliberately not included any inter-rater reliability metrics in Quirkos. Although Quirkos Cloud now allows super-simple project sharing, so a team can code qualitative data simultaneously where-ever they are located, we believe that in most cases it is methodologically inappropriate to use quantitative statistics to measure IRR. Instead, we have tools that show the work done by different coders, so you can have a side-by-side comparison of their coding, helping you to see each time the coding is different and showing you the text so you can understand why.
If you do want interrater statistical tests, Quirkos lets you export your coded data as CSV spreadsheet files, so you can bring it into SPSS or R, where you can run the correct tests for your data (it may not be just Cohen’s Kappa!) and control all the parameters. I’ve always taken the opinion that qualitative statistics should only be done in proper statistical software, by researchers that understand the maths, assumptions and how to interpret the result properly.
If you want to download Quirkos, and try software designed by qualitative researchers for a pure qualitative approach, you can trial the full version for free. It aims to make qualitative analysis software visual and engaging, and makes collaborating and sharing projects easy as well.
References
Armstrong, D., Gosling, A., Weinman, J., Martaeu, T., 1997. The place of inter-rater reliability in qualitative research: an empirical study. Sociology, V31:3, pp597-607 https://www.researchgate.net/publication/240729253_The_Place_of_Inter_Rater_Reliability_in_Qualitative_Research_An_Empirical_Study
Gwet, L., 2011. Agreement Coefficients for Nominal Ratings: A Review. Handbook of Inter-Rater Reliability, 4th Edition. https://www.agreestat.com/book4/9780970806284_chap2.pdf
MacDonald, N., Schoenebeck, S., Forte, A., 2019. Reliability and Inter-rater Reliability in Qualitative Research: Norms and Guidelines for CSCW and HCI Practice. ACM Trans. Graph., Vol. X, No. X, 39. https://andreaforte.net/McDonald_Reliability_CSCW19.pdf
Viera, A., Garrett, J., 2005. Understanding Interobserver Agreement: The Kappa Statistic. Family Medicine, Vol37:5. https://www1.cs.columbia.edu/~julia/courses/CS6998/Interrater_agreement.Kappa_statistic.pdf
McHugh, M., 2012, Interrater reliability: the kappa statistic. Biochemia Medica Vol22:3, pp276-82. https://www.researchgate.net/publication/232646799_Interrater_reliability_The_kappa_statistic/link/5beb1274a6fdcc3a8dd46045/download